The Visual Information laborartory group got together for their annual Summer BBQ last week in Brandon Hill Park, and enjoyed some rare sunshine!
Category: Project
Posts that refer to projects, consortia or collaborations are assigned to this category.
Westminster Policy Forum
We are pleased to announce that Professor Dave Bull has been invited to speak at the Westminster Policy Forum, Next Steps for the Western Gateway
Westminster Social Policy Forum & Policy Forum for Wales keynote seminar: Priorities for economic development and investment in the South Wales and Western England region will be taking place on the 21 June.
This event will evaluate the most recent advancements and future strategies for the South Wales and Western England area. It presents a platform to delve into crucial topics during a period of increased policy attention leading up to the General Election. Attendees will review the progress made thus far and set out priorities for the future to accomplish the goals of the region’s cross-border partnership. These goals include drawing in more private investments into Western England and South Wales, achieving sustainable economic growth, and enhancing coordination and cooperation among local authorities within the region.
Key focuses will include economic development and investment priorities in the South Wales and Western England area. The conference will also explore ways to advance innovation in the region and determine the next steps towards positioning the area as a frontrunner in achieving net zero goals and promoting low carbon energy. The agenda will address the necessary policies and the roles of key stakeholders going forward to facilitate increased financial investments in the region.
Dave Bull, Director of MyWorld will be speaking alongside Director of GW4‘s Joanna Jenkinson, on the theme of Supporting future regional growth, and will be discussing supporting career opportunities, employment and capabilities across the region, examining plans for the UK’s first pan-national Cyber Super Cluster, building upon the region’s digital innovation strengths in AI, 5G, computing and robotics and establishing a coordinated approach and long-term funding for skills development | strengthening
collaboration within the third sector.
IBC 2022 Innovation Award win for UK 5G Edge-XR project
The University of Bristol’s Visual Information Laboratory, part of Bristol Vision Institute is a member of a consortium led by BT Sport that has won the IBC 2022 Innovation Award in the Content Everywhere category. The consortium beat outstanding shortlisted entries from PGA TOUR and SKY Sports. The work resulted from the innovate UK 5G Edge-XR project which can viewed on YouTube.

In collaboration with local start-up, Condense Reality, who have developed a volumetric video capture rig, which captures the 3D essence of people in real time and facilitates digital transportation to a different location, the project enables users to watch a holographic view of the live action, replaying the footage via a game engine from any angle in the comfort of their home.
The experience relies on the speed and low latency of 5G connectivity combined with edge of network computing, to move some of processor load associated with volumetric rendering away from the devices, enabling experiences that have never been seen before.
The University of Bristol’s work in the project, associated with advanced video compression and rendering of volumetric content, led by Andrew Calway, David Bull, Aaron Zhang and Angeliki Katsenou is now being further developed through the MyWorld programme, collaboratively with BT and Condense Reality.
Further information
Condense; Salsa Sound; THE GRID FACTORY; University of Bristol; DANCE EAST
MyWorld PhD Scholarship: Low Light Fusion and Autofocus for Advanced Wildlife Coverage
About the Project
Natural History filmmaking presents many challenges. For example, filming in low light or using modalities such as infra-red can result in noisy, low-resolution images, or can suffer from poor contrast range and colours. Also, the camera sensor is normally operating with high ISO levels and hence has wide aperture and extremely shallow depth of field.
This project will enable production workflow to push the boundaries of what is possible in terms of new image acquisition and processing methods for telling stories of the natural world. The project will look at image-based approaches to understanding and explaining the natural world, for example by combining multiple imaging modalities such as visible and infra-red. It will investigate means of autofocus for low light content using spectral, spatial, or other image processing methods to control the focus action. Machine learning methods to estimate focus from blur after training will also be explored.
Launched in April 2021, MyWorld is a brand-new five-year programme, the flagship for the UK’s creative technology sector, and is part of a UK-wide exploration into devolved research and development funding (UKRI video). Led by the University of Bristol, MyWorld will position the South West as an international trailblazer in screen-based media. This £46m programme will bring together 30 partners from Bristol and Bath’s creative technologies sector and world-leading academic institutions, to create a unique cross-sector consortium. MyWorld will forge dynamic collaborations to progress technological innovation, deliver creative excellence, establish, and operate state of the art facilities, offer skills training and drive inward investment, raising the region’s profile on the global stage.
URL for further information: http://www.myworld-creates.com/
Candidate Requirements
Applicants must hold/achieve a minimum of a master’s degree (or international equivalent) in a relevant discipline. Applicants without a master’s qualification may be considered on an exceptional basis, provided they hold a first-class undergraduate degree. Please note, acceptance will also depend on evidence of readiness to pursue a research degree.
If English is not your first language, you need to meet this profile level:
Profile E
Further information about English language requirements and profile levels.
Basic skills and knowledge required
Essential: Excellent analytical skills and experimental acumen.
Desirable: A background understanding in one or more of the following:
- Image processing
- Artificial intelligence/Machine learning/Deep learning
- Computational Imaging / Computational Photography
Application Process
- All candidates should submit a full CV and covering letter to myworldrecruitment@myworld-creates.com (FAO: Professor David R. Bull).
- Formal applications for PhD are not essential at this stage, but can be submitted via the University of Bristol homepage (clearly marked as MyWorld funded): https://www.bristol.ac.uk/study/postgraduate/apply/
- A Selection Panel will be established to review all applications and to conduct interviews of short-listed candidates.
- This post remains open until filled.
For questions about eligibility and the application process please contact SCEEM Postgraduate Research Admissions sceem-pgr-admissions@bristol.ac.uk
Funding Notes
Stipend at the UKRI minimum stipend level (£16,062 p.a. in 2022/23) will also cover tuition fees at the UK student rate. Funding is subject to eligibility status and confirmation of award.
To be treated as a home student, candidates must meet one of these criteria:
- be a UK/EU national (meeting residency requirements)
- have settled status
- have pre-settled status (meeting residency requirements)
- have indefinite leave to remain or enter.
Analysis of Coral using Deep Learning
Tilo Burghardt, Ainsley Rutterford, Leonardo. Bertini, Erica J. Hendy, Kenneth Johnson, Rebecca Summerfield
Animal biometric systems can also be applied to the remains of living beings – such as coral skeletons. These fascinating structures can be scanned via 3D tomography and made available to computer vision scientists via resulting image stacks.
In this project we investigated the efficacy of deep learning architectures such as U-Net on such image stacks in order to find and measure important features in coral skeletons automatically. One such feature is constituted by growth bands of the colony, which are extracted/approximated by our system and superimposed on a coral slice in the image below. The project provides a first proof-of-concept that machines can, given sufficiently clear samples, perform similarly to humans in many respects when identifying associated growth and calcification rates exposed from skeletal density-banding. This is a first step towards automating banding measurements and related analysis.
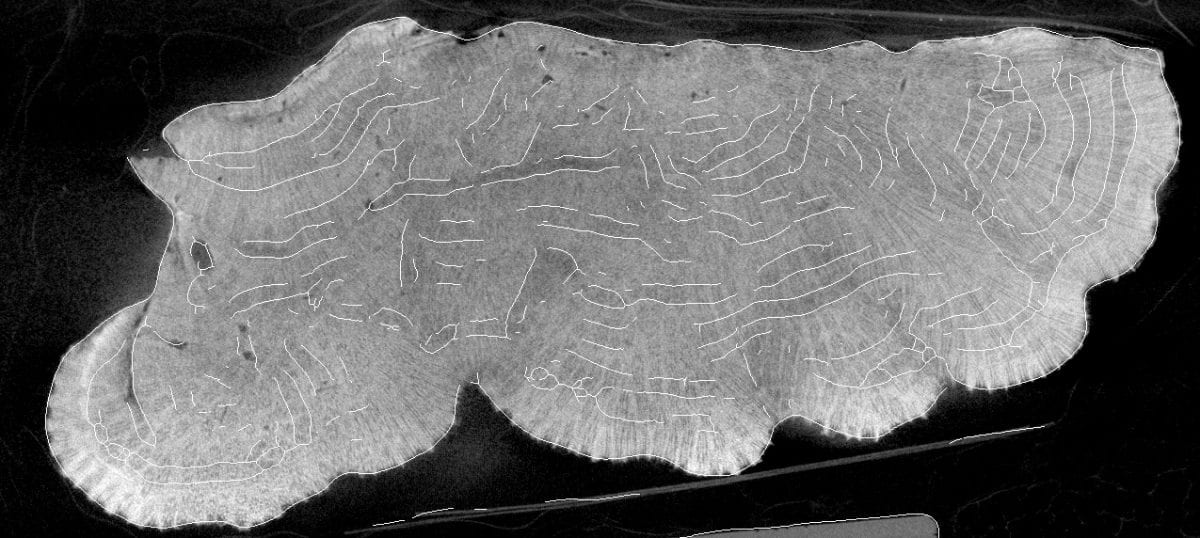
This work was supported by NERC GW4+ Doctoral Training Partnership and is part of 4D-REEF, a Marie Sklodowska-Curie Innovative Training Network funded by European Union Horizon 2020 research and innovation programme under the Marie Sklodowska-Curie grant agreement No. 813360.
Code Repository at https://github.com/ainsleyrutterford/deep-learning-coral-analysis
Aerial Animal Biometrics
Tilo Burghardt, Will Andrew, Colin Greatwood
Traditionally animal biometric systems represent and detect the phenotypic appearance of species, individuals, behaviors, and morphological traits via passive camera settings – be this camera traps or other fixed camera installations.
In this line of work we implemented for the first time a full biometric pipeline onboard a autonomous UAV in order to gain complete autonomous agency that can be used to adjust acquisition scenarios to important settings such as individual identification in freely moving herds of cattle.
In particular, we have built a computationally-enhanced M100 UAV platform with an on-board deep learning inference system for integrated computer vision and navigation able to autonomously find and visually identify by coat pattern individual HolsteinFriesian cattle in freely moving herds.We evaluate the performance of components offline, and also online via real-world field tests of autonomous low-altitude flight in a farm environment. The proof-of-concept system is a successful step towards autonomous biometric identification of individual animals from the air in open pasture environments and in-side farms for tagless AI support in farming and ecology.
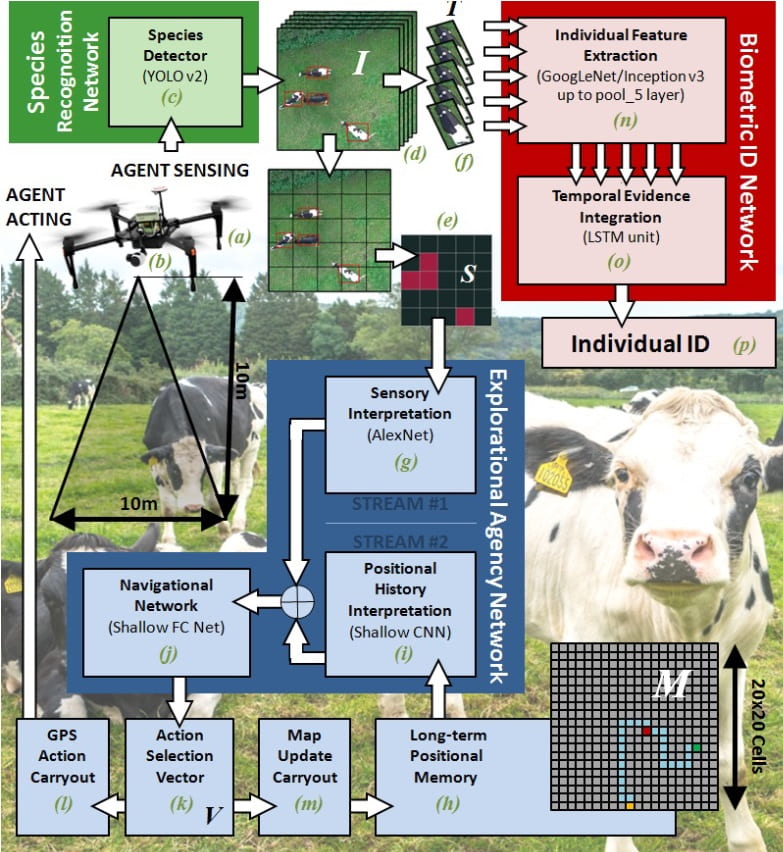
This work was conducted in collaboration with Farscope CDT, VILab and BVS.
Related Publications
W Andrew, C Greatwood, T Burghardt. Aerial Animal Biometrics: Individual Friesian Cattle Recovery and Visual Identification via an Autonomous UAV with Onboard Deep Inference. 32nd IEEE/RSJ International Conference on Intelligent Robots and Systems (IROS), pp. 237-243, November 2019. (DOI:10.1109/IROS40897.2019.8968555), (Arxiv PDF)
W Andrew, C Greatwood, T Burghardt. Deep Learning for Exploration and Recovery of Uncharted and Dynamic Targets from UAV-like Vision. 31st IEEE/RSJ International Conference on Intelligent Robots and Systems (IROS), pp. 1124-1131, October 2018. (DOI:10.1109/IROS.2018.8593751), (IEEE Version), (Dataset GTRF2018), (Video Summary)
W Andrew, C Greatwood, T Burghardt. Visual Localisation and Individual Identification of Holstein Friesian Cattle via Deep Learning. Visual Wildlife Monitoring (VWM) Workshop at IEEE International Conference of Computer Vision (ICCVW), pp. 2850-2859, October 2017. (DOI:10.1109/ICCVW.2017.336), (Dataset FriesianCattle2017), (Dataset AerialCattle2017), (CVF Version)
HS Kuehl, T Burghardt. Animal Biometrics: Quantifying and Detecting Phenotypic Appearance. Trends in Ecology and Evolution, Vol 28, No 7, pp. 432-441, July 2013.
(DOI:10.1016/j.tree.2013.02.013)