Tilo Burghardt, Ainsley Rutterford, Leonardo. Bertini, Erica J. Hendy, Kenneth Johnson, Rebecca Summerfield
Animal biometric systems can also be applied to the remains of living beings – such as coral skeletons. These fascinating structures can be scanned via 3D tomography and made available to computer vision scientists via resulting image stacks.
In this project we investigated the efficacy of deep learning architectures such as U-Net on such image stacks in order to find and measure important features in coral skeletons automatically. One such feature is constituted by growth bands of the colony, which are extracted/approximated by our system and superimposed on a coral slice in the image below. The project provides a first proof-of-concept that machines can, given sufficiently clear samples, perform similarly to humans in many respects when identifying associated growth and calcification rates exposed from skeletal density-banding. This is a first step towards automating banding measurements and related analysis.
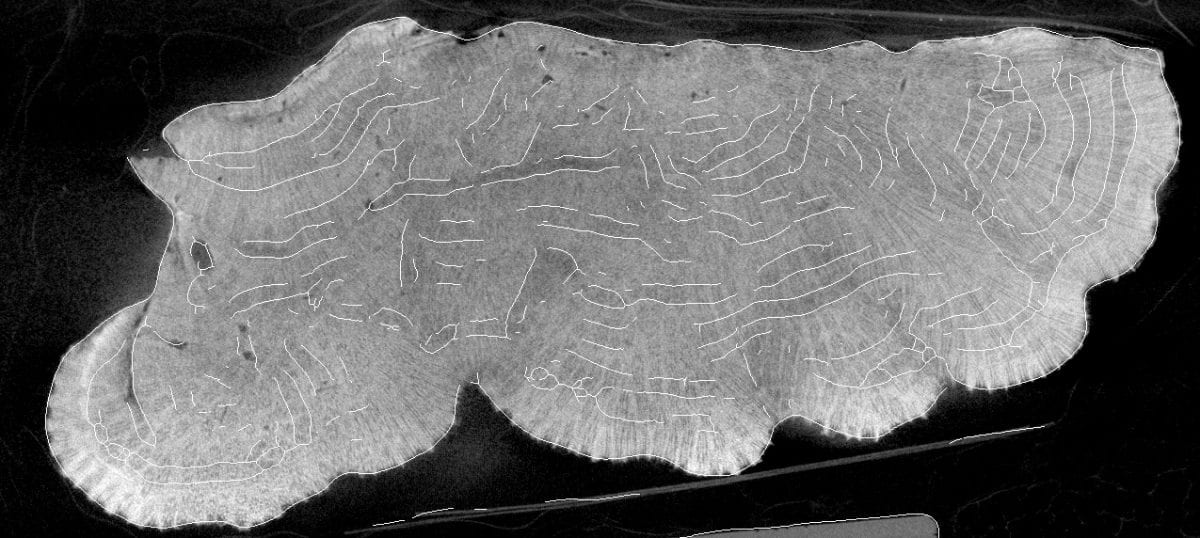
This work was supported by NERC GW4+ Doctoral Training Partnership and is part of 4D-REEF, a Marie Sklodowska-Curie Innovative Training Network funded by European Union Horizon 2020 research and innovation programme under the Marie Sklodowska-Curie grant agreement No. 813360.
Code Repository at https://github.com/ainsleyrutterford/deep-learning-coral-analysis