Tilo Burghardt, Will Andrew, Colin Greatwood
Traditionally animal biometric systems represent and detect the phenotypic appearance of species, individuals, behaviors, and morphological traits via passive camera settings – be this camera traps or other fixed camera installations.
In this line of work we implemented for the first time a full biometric pipeline onboard a autonomous UAV in order to gain complete autonomous agency that can be used to adjust acquisition scenarios to important settings such as individual identification in freely moving herds of cattle.
In particular, we have built a computationally-enhanced M100 UAV platform with an on-board deep learning inference system for integrated computer vision and navigation able to autonomously find and visually identify by coat pattern individual HolsteinFriesian cattle in freely moving herds.We evaluate the performance of components offline, and also online via real-world field tests of autonomous low-altitude flight in a farm environment. The proof-of-concept system is a successful step towards autonomous biometric identification of individual animals from the air in open pasture environments and in-side farms for tagless AI support in farming and ecology.
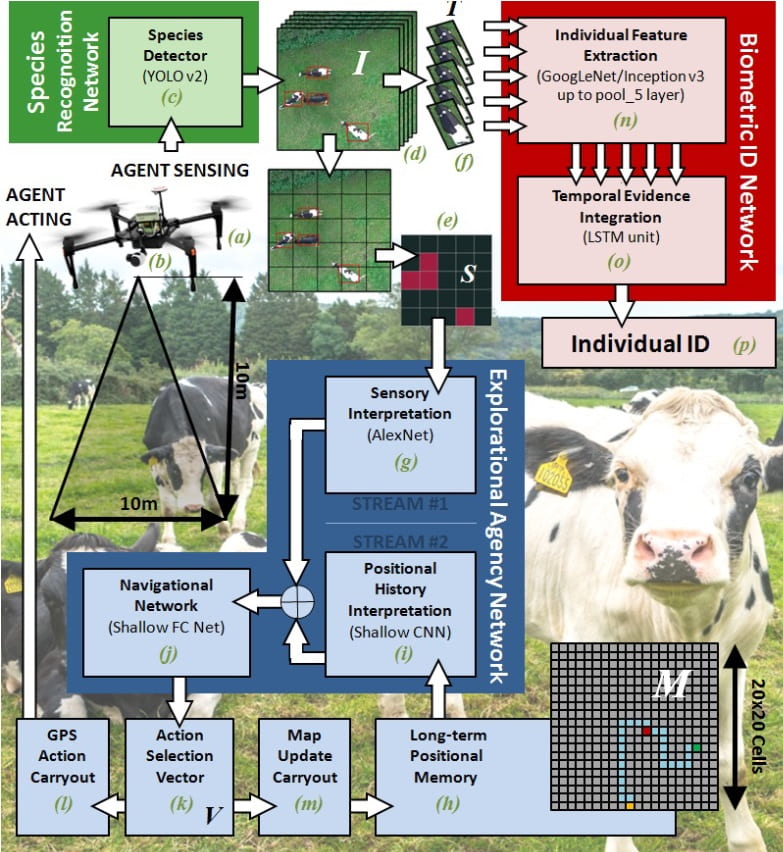
This work was conducted in collaboration with Farscope CDT, VILab and BVS.
Related Publications
W Andrew, C Greatwood, T Burghardt. Aerial Animal Biometrics: Individual Friesian Cattle Recovery and Visual Identification via an Autonomous UAV with Onboard Deep Inference. 32nd IEEE/RSJ International Conference on Intelligent Robots and Systems (IROS), pp. 237-243, November 2019. (DOI:10.1109/IROS40897.2019.8968555), (Arxiv PDF)
W Andrew, C Greatwood, T Burghardt. Deep Learning for Exploration and Recovery of Uncharted and Dynamic Targets from UAV-like Vision. 31st IEEE/RSJ International Conference on Intelligent Robots and Systems (IROS), pp. 1124-1131, October 2018. (DOI:10.1109/IROS.2018.8593751), (IEEE Version), (Dataset GTRF2018), (Video Summary)
W Andrew, C Greatwood, T Burghardt. Visual Localisation and Individual Identification of Holstein Friesian Cattle via Deep Learning. Visual Wildlife Monitoring (VWM) Workshop at IEEE International Conference of Computer Vision (ICCVW), pp. 2850-2859, October 2017. (DOI:10.1109/ICCVW.2017.336), (Dataset FriesianCattle2017), (Dataset AerialCattle2017), (CVF Version)
HS Kuehl, T Burghardt. Animal Biometrics: Quantifying and Detecting Phenotypic Appearance. Trends in Ecology and Evolution, Vol 28, No 7, pp. 432-441, July 2013.
(DOI:10.1016/j.tree.2013.02.013)