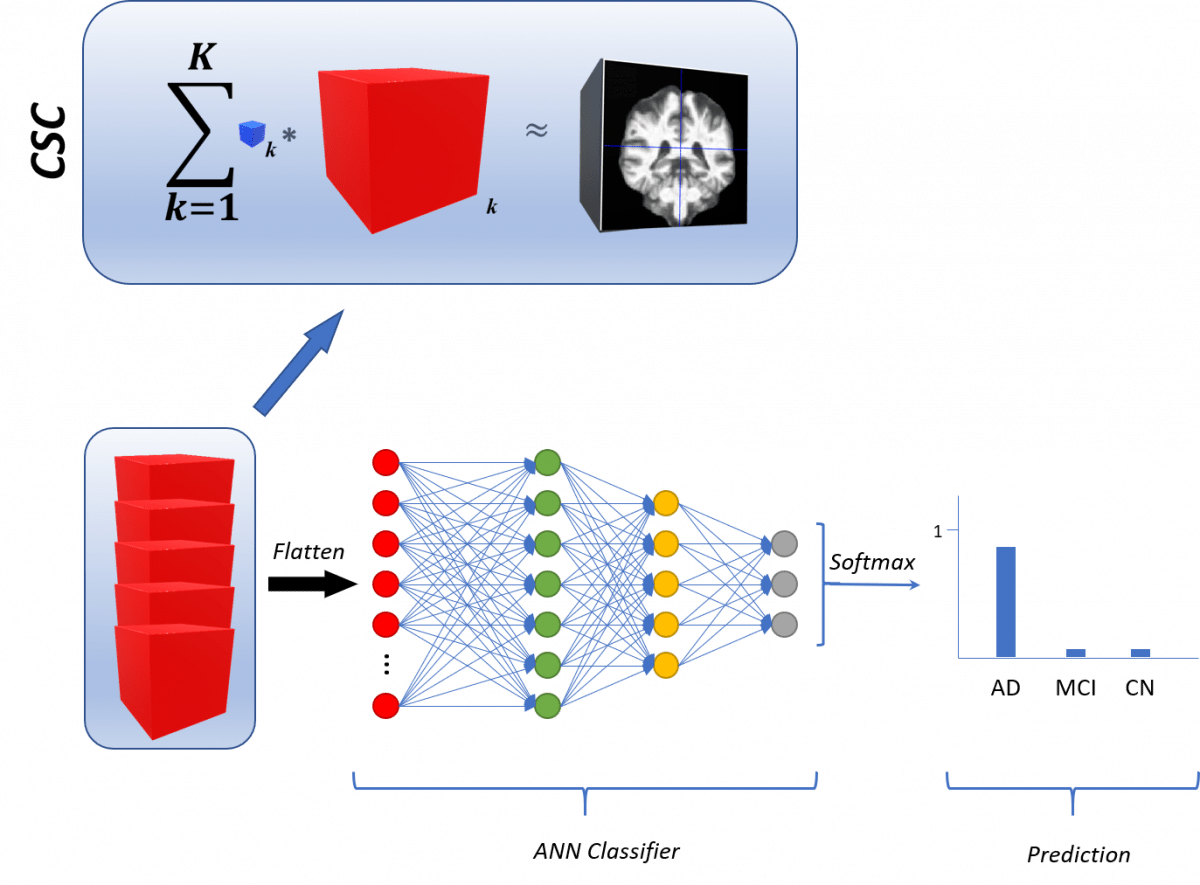
The learning and selection of features are leveraged to the Convolutional Sparse Coding approach, which is applied to the 3-dimensional MRIs in the dataset. They can be fed to any classifier, a fully connected neural network is shown here. The output corresponds to the prediction of the model for a given brain scan.
Diagnosis in medicine can be further aided by the automated inspection of structural images from the various body parts. Thus, the automated algorithm should be able to learn features from the images such that they will further aid in the accurate distinction of the disease, if present, from the image. This process can be done end-to-end using state-of-the-art techniques such as Convolutional Neural Networks (CNNs). In this work, we propose an alternative algorithm in which the diagnosis is performed as a two-step process. First, we learn the features from the 3-dimensional data, structural Magnetic Resonance Imaging (MRI) scans via Convolutional Sparse Coding (CSC). The features learned are then fed into some classifier –even CNNs—to fit a model able to differentiate the classes of interest, which correspond to the different diagnosis the patient could have for a particular disease