
- This event has passed.
VILSS: Transductive Transfer Learning for Computer Vision
Tuesday 21, April, 2015 @ 14:00 - 15:00
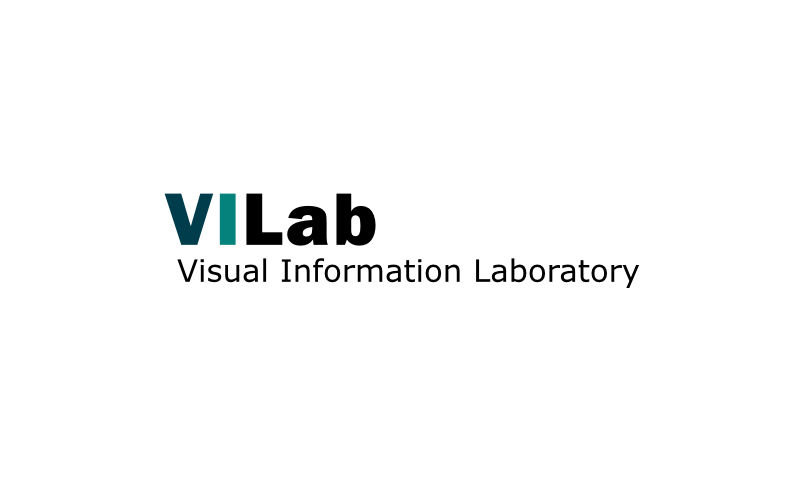
Teo de Campos, University of Surrey
One of the ultimate goals of the open ended learning systems is to take advantage of previous experience in dealing with future problems. We focus on classification problems where labelled samples are available in a known problem (the source domain), but when the system is deployed in the target dataset, the distribution of samples is different. Although the number of classes and the feature extraction method remain the same, a change of domain happens because there is a difference between the typical distribution of data of source and target samples. This is a very common situation in computer vision applications, e.g., when a synthetic dataset is used for training but the system is applied on images “in the wild”. We assume that a set of unlabelled samples is available target domain. This constitutes a Transductive Transfer Learning problem, also known as Unsupervised Domain Adaptation. We proposed to tackle this problem by adapting the feature space of the source domain samples, so that their distribution becomes more similar to that of the target domain samples. Therefore a classifier re-trained on the updated source space can give better results on the target samples. We proposed to use a pipeline which consists of three main components: (i) a method for global adaptation of the marginal distribution of the data using Maximum Mean Discrepancy; (ii) a sample-based adaptation method, which translates each source sample towards the distribution of the target samples; (iii) a class-based conditional distribution adaptation method. We conducted experiments on a range of image classification and action recognition datasets and showed that our method gives state-of-the-art results.